- Build robust and generalizable models
- Develop unbiased models that provide the most valuable insights
- Keep up with AI trends and innovations
Are you frustrated by the never ending cycle of machine learning experiments?
- Uncertain about the best approaches for your application?
- Worried about mitigating the ethical concerns of AI?
- Struggling to keep up with the rapidly advancing field?
Boost investor confidence and streamline AI development
Here are three ways I can help...
Computer Vision Insights Newsletter
For founders, executives, managers, researchers, and engineers who want to stay abreast of the latest advances
Strategy Session
Practical advice to move your project forward
Team Workshop
A 90 minute private workshop to unlock new possibilities for your AI product development
Hi, I’m Heather Couture, and I accelerate computer vision projects with evidence-driven model development
While working with a variety of organizations on computer vision projects, I’ve witnessed the long and iterative development cycles. Every dataset is different, so it does take some experimentation to get a robust model.
But some of this experimentation could be reduced by following three key principles:
- Identifying sources of variation or other data challenges – domain experts are essential for this
- Understanding prior work – a literature review can find candidate solutions and unresolved challenges
- Validating early and often to reveal failure modes – essential for making improvements
Model development progresses much faster and smoother when you understand the challenges in your dataset and can try solutions that have worked for others on similar data. And proper validation can focus your efforts to improve your models and help you recognize major obstacles earlier.
I guide organizations in following these principles. Given the unique characteristics of a dataset, I help them identify the best path to robust and generalizable models, enabling them to take their products to market sooner.
Organizations I've Worked With
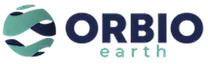
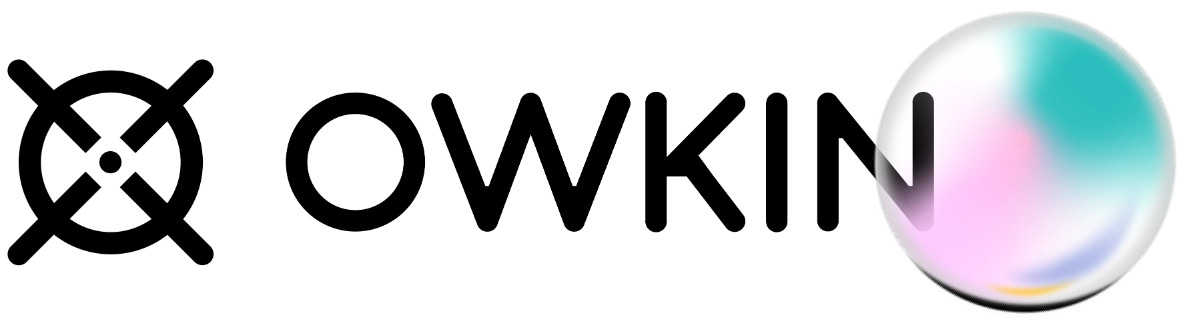
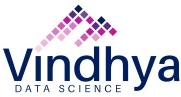
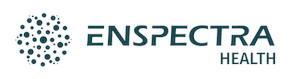
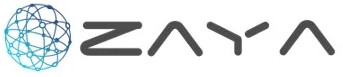
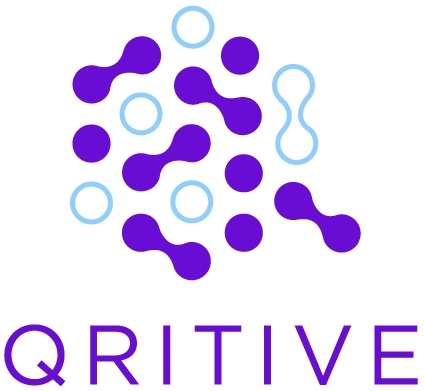
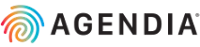
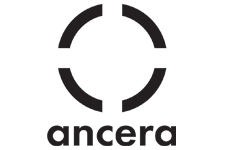

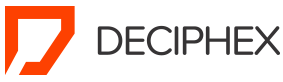
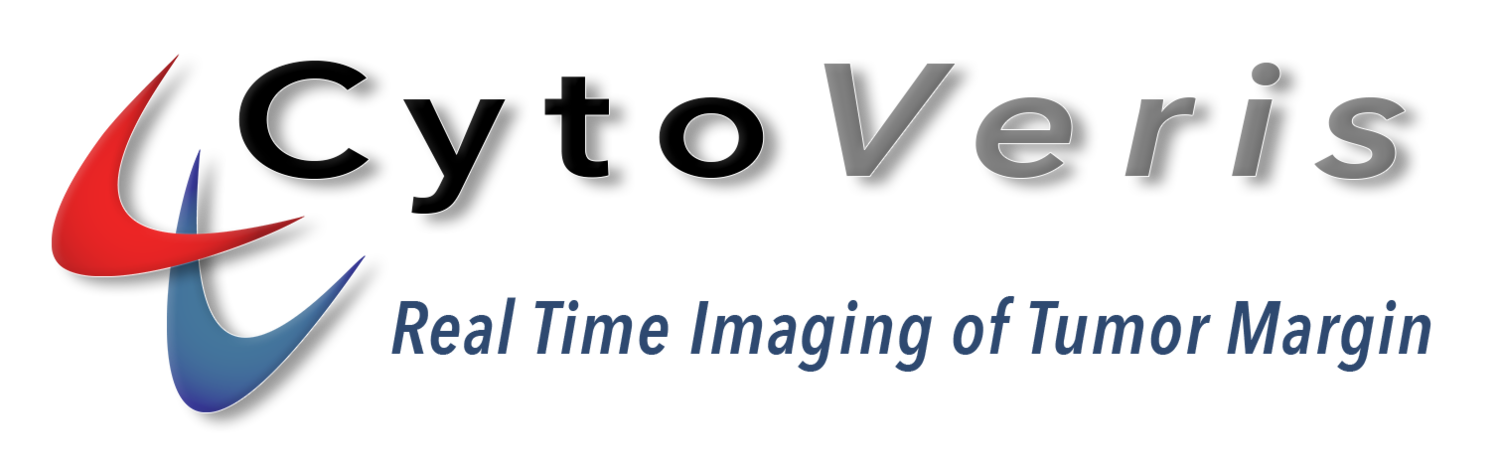
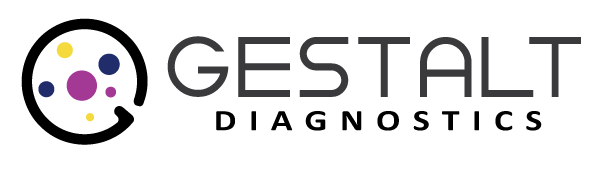
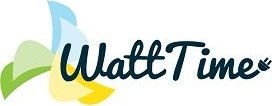
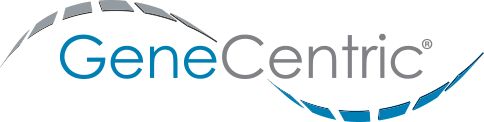
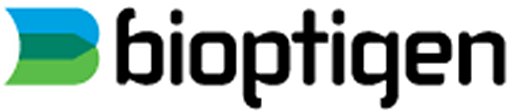
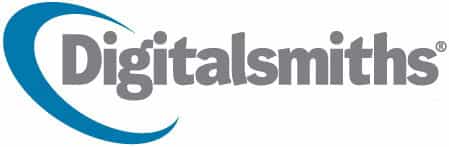
Why work with me?
Proven Track Record
Accelerated CV/ML projects for more than 15 clients – mostly seed or early stage startups.
Domain Knowledge
Heavily focused on microscopy and satellite imagery, providing deep insights and the ability to collaborate across disciplines.
State-of-the-Art Expertise
20 years of experience in CV/ML
PhD in Computer Science
15+ peer-reviewed publications