Cancer Detection in Autofluorescence Imagery During Surgery
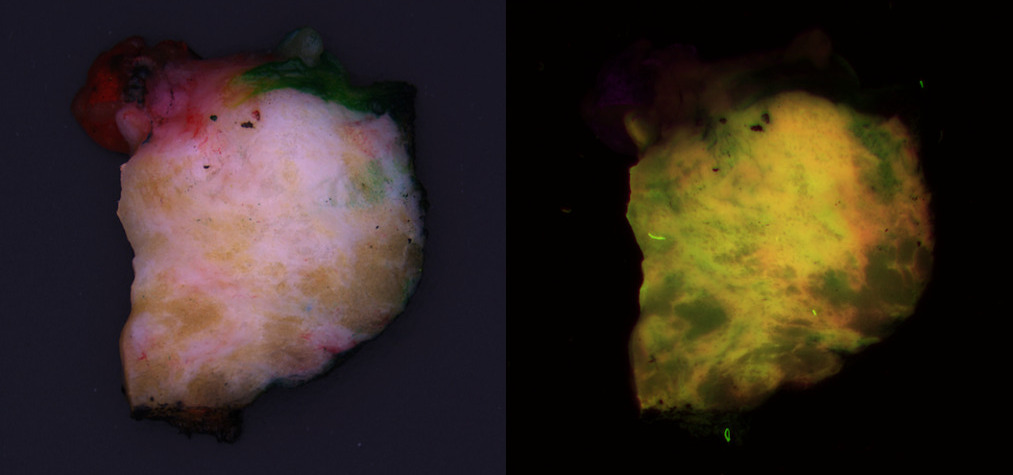
Project Details
Problem: Surgeons try to conserve as much healthy tissue as possible, while excising all tumor tissue. However, distinguishing between the two during surgery is challenging. For a definitive call, the tissue must be processed for histological analysis. CytoVeris is developing an ultraviolet-excited autofluorescence platform to image excised tissue during surgery and highlight any regions that are likely to be cancerous.
Solution: I assisted CytoVeris in training machine learning models to distinguish cancer from healthy breast tissue in multispectral autofluorescence images. Pathologist annotations were obtained from the corresponding H&E image and were spatially referenced with autofluorescence images of the tissue. Some of the technical challenges included the diversity and mixed heterogeneity of tissue samples, and the precision of the image to H&E registration, both of which can result in some degree of label uncertainty. We compared a variety of different methods for feature extraction and classification. The model results also provided feedback for data cleaning and model improvement efforts. As lighting and optical techniques are improved in new iterations of the imaging device, the models continually became more robust.
OncoSIGHT AI
Archives of Pathology & Laboratory Medicine: AI-Powered Biomolecular-Specific and Label-Free Multispectral Imaging Rapidly Detects Malignant Neoplasm in Surgically Excised Breast Tissue Specimens
Press Release